2023. 4. 14. 12:36ㆍ공간농업, 농업공간/Multifunctional agriculture
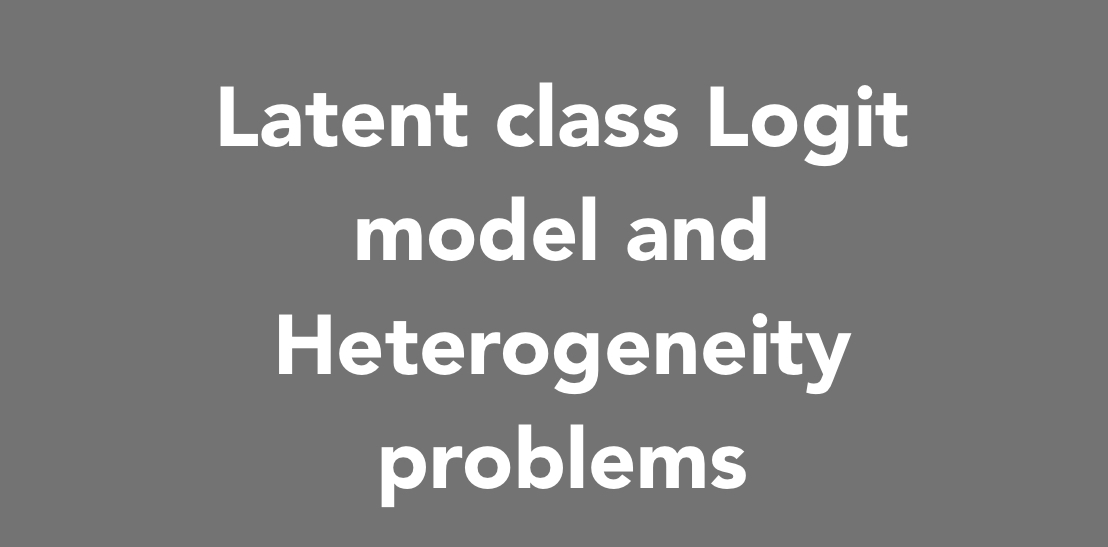
4.2.2 Latent class model and Heterogeneity problems
Meanwhile, the estimation in the above section has a problem not considering heterogeneity in preferences of environmental attributes. Recent CE studies on MFA found heterogeneous preferences. For example, Dominguez-Torreiro and Solino (2011) analyzed CE data on multifunctional rural development policies using random parameter logit (mixed logit) to account for unobserved taste heterogeneity. Sangkapitux et al. (2017) found heterogeneity in preferences of MFA using latent class logit model. Therefore, it is necessary to apply a model that considers validation and heterogeneity to the results of this study. In this chapter, the latent class conditional logit (LCL) model that can be applied to the conditional logit model is applied to examine whether there is heterogeneity in the model, and to separate or remove the heterogeneous latent class to verify the model.
The LCL model extends the conditional logit model by incorporating a discrete representation of unobserved preference heterogeneity. Algebraically, the LCL likelihood function is a finite mixture of classes different conditional logit likelihood function (Yoo, 2020).
LCL extends the conditional logit by incorporating discrete representations of unobserved preference heterogeneity among decision makers. LCL also assumes that there are distinct types or classes of decision makers, and that each class makes choices consistent with its own clogit model using a utility coefficient vector β c . Assume that the probability that a decision maker n belongs to class c is given by a fractional multinomial logit specification (Yoo, 2019).

By Yoo’s multinomial logit specification (5.4) where zn is a row vector of decision maker n’s characteristics and the usual constant regressor (i.e. 1); θc is a conformable column vector of membership model coefficients for class c, with θc normalized to 0 for identification; and γ = (θ1,θ2,…,θC-1 ) denotes a collection of the C -1 identified membership coefficient vectors. Under LCL, the joint likelihood of decision maker n’s choices is given by

where B=(β1, β2,…, βC) denote a collection of the C utility coefficient vectors, and each Pn(βc) is obtained by evaluating general conditional logit model.
It is necessary to determine whether there is heterogeneity in the conditional logit result in Table 5.10, and LCL analysis was performed to find out how many potential classes are on it. Table 5.13 shows the estimated coefficient of each variable for each latent class. As a result of the analysis, it was found that there are two latent classes and there is heterogeneity. An appropriate number of potential classes can be determined according to the result of the Bayesian information criterion (BIC). In two cases, the BIC result is 1935.2, whereas in the case of three, the BIC result increases to 1957.9. The smaller the value, the more appropriate the class.
Among the two classes, Class 1 occupies 71.9% of the total sample, and Class 2 shows that the hierarchy can be separated by 28.1%, and there is heterogeneity in nature and environment, history and culture, and public activity variables.
According to the LCL estimation result, the value of MOA can estimate the optimal value of the two latent classes. Through maximizing loglikelihood using EM algorithm, Class 1, Class 2, and Share 1 correspond to (β1, β2,…, βC) and θ1 respectively for equations (5.4) and (5.5) above. Table 5.14 is obtained as a result of estimating the five random coefficients variables involving heterogeneity presented below in Table 5.13 and cost variables.
Table 5.13 Estimation result of LCL
Variables | 2 classes | 3 classes | |||
Class1 | Class2 | Class1 | Class2 | Class3 | |
Nature and environment | 0.084 | -0.098 | 0.163 | -0.036 | 0.077 |
History and culture | 0.019 | 0.167 | 0.047 | 0.078 | -0.002 |
Landscape | 0.031 | 0.021 | -0.014 | 0.005 | 0.101 |
Public activity | 0.048 | -0.047 | 0.028 | -0.018 | 0.098 |
Cost | -0.000 | 0.001 | -0.000 | 0.000 | -0.001 |
Class Share | 0.719 | 0.281 | -0.371 | 0.295 | 0.334 |
Result of BIC | 1935.2 | 1957.9 | |||
Log likelihood | -928.4 | -928.3 |
As a result of LCL estimation, in Class 2, the values of nature and environment, history and culture For MOA variables were significant, but the values of other variables were not significant. In addition, the coefficient value of the nature and environment variable obtained a significant probability value, but it was estimated as a negative sign even it should be positive, and it seems that the results caused by the respondent or judgment error were included in Class 2.
Table 5.14 The estimation value of LCL MLE
Variables (ML) | Class1 | Class2 | Value of class 1 (USD) | |
Nature and environment | 0.083509*** | -0.09932*** | 0.310 | |
History and culture | 0.019036*** | 0.168824*** | 0.070 | |
Landscape | 0.03054*** | 0.021218 | 0.113 | |
Public activity | 0.04839*** | -0.04729 | 0.179 | |
Cost | -0.00027*** | 0.000526*** | ||
Class Share | 0.719 | 0.281 | ||
Share1 | Constant | 0.941507*** | ||
Log likelihood | -928.4 | |||
Note: *** is ρ -value parameter below 1%.
On the other hand, the result of Class 1 occupies 71.9% of the total sample, and significant values were estimated for the four attributes of MOA and also the cost variable for which the value was to be estimated in this chapter.
As a result of estimation by the LCL model in Table 5.14, it showed the largest change in the value size. It was about 25% of the value estimated by the conditional logit model. The value of natural environment resources was estimated to be the highest at USD 0.310, followed by public activity at USD 0.179, landscape at USD 0.113 and history and cultural resources at USD 0.07 at the lowest estimate. In the ranking change of value, the value of history and culture resources ranked second in the conditional logit model in Table 5.10, but LCL showed the lowest value. It means that the selection of respondent for the history and culture of the Class1 and Class2 layers are very different and has heterogeneity.
As a result, it can be seen that the conditional logit model has heterogeneity in the existence value of MOA, but in fact, people evaluate it as a lower value than the researcher’s expectation. In order to further clarify the existence value of MOA, it is necessary to construct a clear questionnaire to reduce heterogeneity and to secure a larger number of samples to analyse meaningful LCL results between classes.
'공간농업, 농업공간 > Multifunctional agriculture' 카테고리의 다른 글
농업의 다원적 기능이란 (What is multifunctionality of agriculture) (0) | 2023.12.15 |
---|---|
(pdf) Multifunctional Agriculture in EU (Cases) (0) | 2022.12.27 |
(pdf) Difference between Multifunctional agriculture and Multifunctionality of a (0) | 2022.12.27 |
(pdf) Multifunctional agriculture functions and effects (0) | 2022.12.27 |
Multifunctional agriculture definition (0) | 2022.12.27 |